How Banks Use Data Analytics financial services landscape, the use of data analytics has become a cornerstone for improving customer experiences in the banking sector. As consumers demand faster, more personalized, and seamless interactions with their banks, leveraging data analytics offers institutions the ability to stay competitive while driving customer satisfaction. This article delves into how banks are harnessing the power of data analytics to enhance the customer experience, from personalization and proactive service offerings to fraud prevention and operational efficiency.
1. Introduction: The Importance of Data Analytics in Banking
Data analytics has revolutionized industries across the globe, and the banking sector is no exception. The modern customer expects more than just traditional banking services; they demand an experience that is tailored, responsive, and consistent across all touchpoints. As a result, banks are increasingly turning to data analytics to gain deeper insights into customer preferences, behaviors, and needs, thereby allowing them to deliver a more personalized and efficient service.
At its core, data analytics enables banks to extract valuable insights from vast amounts of structured and unstructured data. This data can range from transactional information and customer interactions to social media activity and external economic factors. By analyzing this data, banks can make data-driven decisions that improve everything from product offerings to customer support.
2. Personalization: Tailoring Banking Services to Individual Needs
One of the most powerful ways that data analytics is transforming the customer experience in banking is through personalization. Consumers today expect services that reflect their unique needs and preferences. With the help of data analytics, banks can create personalized experiences that not only meet but exceed customer expectations.
2.1 Customer Segmentation and Targeting
By using advanced analytics, banks can segment their customer base into distinct groups based on factors such as demographics, behavior, financial goals, and spending habits. This segmentation allows banks to target specific customer needs with tailored products and services, increasing the likelihood of customer satisfaction and retention.
For example, a bank may offer a personalized loan product with specific interest rates to customers who have a history of saving and a high credit score. Alternatively, they might send a targeted message to a customer who frequently travels abroad, offering them a special travel rewards credit card.
2.2 Dynamic Pricing and Offers
Data analytics also allows banks to implement dynamic pricing and promotional offers based on real-time data. By analyzing customer spending patterns and transaction history, banks can identify the most relevant offers for individual customers at the right time. This ensures that promotional offers resonate with the customer, making them feel valued and understood.
For example, if a customer frequently makes large purchases, the bank might offer them an exclusive credit card with a higher limit and additional rewards. Similarly, for a customer who has been saving diligently, the bank could propose investment products that align with their long-term financial goals.
3. Proactive Customer Service: Anticipating Needs Before They Arise
The ability to anticipate customer needs and proactively address potential issues is another key way that banks are using data analytics to enhance the customer experience. Rather than waiting for customers to reach out with problems, banks can identify and resolve issues before they escalate.
3.1 Predictive Analytics for Service Improvement
Predictive analytics, a branch of data analytics that uses historical data to forecast future trends, enables banks to anticipate customer issues and needs. For instance, by analyzing transaction patterns, banks can predict when a customer might need assistance, such as during a large transaction or a period of financial stress.
For example, predictive models can help identify when a customer might be at risk of overdrawing their account or when they are likely to encounter issues with their credit card payment. In such cases, the bank can reach out to offer assistance, suggest solutions, or even offer a temporary credit increase to ease their financial strain.
3.2 Chatbots and AI-Driven Support
Artificial intelligence (AI) and chatbots powered by data analytics are also revolutionizing how banks interact with customers. These AI-powered tools use data to provide instant responses to customer inquiries, improving response times and eliminating the need for customers to wait in long queues.
Moreover, chatbots can learn from past interactions, continuously improving their responses and providing more accurate and relevant solutions. This results in more efficient customer service and a more satisfying experience for users who prefer immediate assistance.
4. Fraud Detection and Security: Building Trust and Safety
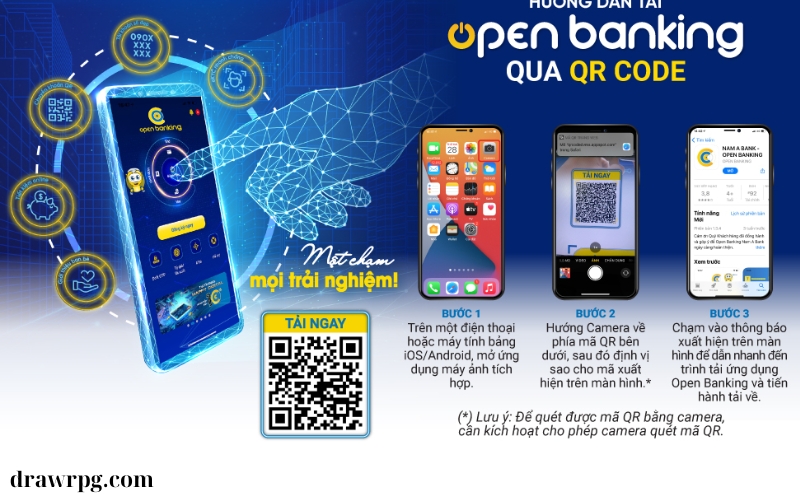
Security and fraud prevention are top priorities for both banks and their customers. Data analytics plays a vital role in identifying fraudulent activities and ensuring that customers’ financial information remains safe. With the help of advanced algorithms and machine learning, banks can detect unusual patterns of behavior that may indicate fraudulent activity.
4.1 Real-Time Fraud Detection
Banks utilize data analytics to monitor transactions in real-time, flagging suspicious activities such as unauthorized transactions or unusual spending patterns. By analyzing vast amounts of transactional data, machine learning algorithms can identify patterns that deviate from the norm and trigger alerts for further investigation.
For example, if a customer’s credit card is suddenly used in a foreign country where they have not previously traveled, the bank can immediately block the transaction and notify the customer for verification. This proactive approach minimizes the risk of fraud and reassures customers that their money is safe.
4.2 Enhancing Cybersecurity with Big Data
Data analytics also helps banks strengthen cybersecurity by continuously monitoring network traffic and identifying potential threats. By analyzing data from multiple sources, such as customer accounts, device fingerprints, and location information, banks can enhance their security protocols and prevent unauthorized access to customer data.
With cyberattacks becoming increasingly sophisticated, the use of big data analytics to detect and thwart these threats is crucial for maintaining customer trust. Banks can leverage advanced anomaly detection techniques to identify potential vulnerabilities and implement countermeasures before they are exploited.
5. Enhancing Operational Efficiency: Streamlining Banking Processes
Beyond customer-facing benefits, data analytics also plays a significant role in improving the internal operations of banks, which indirectly impacts the customer experience. By streamlining banking processes and improving operational efficiency, banks can deliver faster services, lower costs, and better overall experiences.
5.1 Automation of Routine Tasks
Data analytics allows banks to automate many routine tasks, such as processing loan applications, verifying customer identities, or managing transaction records. Automation not only reduces the time required for these tasks but also minimizes the potential for human error, ensuring a smoother and more reliable service for customers.
For example, machine learning algorithms can quickly process loan applications and assess creditworthiness, dramatically reducing the time it takes for customers to receive approval. Similarly, automated systems can handle basic inquiries, freeing up customer service representatives to focus on more complex issues.
5.2 Optimizing Resource Allocation
By analyzing operational data, banks can optimize their resource allocation and improve decision-making. For example, by analyzing customer traffic patterns and transaction volumes, banks can determine the optimal staffing levels at branches or deploy resources to areas where customers are most likely to need assistance.
This data-driven approach to resource management helps banks improve customer service and satisfaction, as they can ensure that the right resources are in place to meet customer needs when and where they arise.